树 树/自由树(tree/free tree)是一个连通的、非循环的无向图
前文 初步阐释了树,以及对于二叉树、AVL树的实现与分析 。 最后简单介绍了红黑树、伸展树、B/B+树,如果有需要/有机会的话补充这三者的实现与分析(好复杂)
树的介绍 树有多种方式定义,其中一种自然的方式是采用递归 一棵树是一些点的集合,这个集合也能是空集; 若不为空集,树就由称作跟root的节点r,以及0到N个的非空(子)树组成,子树的每一棵的跟都有来自r的一条有向边连接 在递归定义中,我们认为“一棵树是N个节点和N-1跳边的集合,其中一个节点为根root”
树与节点 根节点:最初的节点,被称为根或根节点 Root/Root Node 叶/外节点:没有子节点的的点 Leaf/External Node 内节点:非叶节点的其他节点 Internal Node
深度Depth:从根到节点的简单路径的长度 高度Height:一棵树的最大深度,对于任意节点ni,ni的深度到一片树叶的最远路径长 Degree 根节点的子节点数目
节点祖先 Ancestor of a node:从根节点到指定节点的唯一简单路径上的任何节点 后代 Descendant: 如果A是B的祖先节点,则B是A的后代节点
每个节点都是自己的Ancestor和Descendant
父节点 Parent:从根节点到指定节点的简单路径上的最后一条边的那个点,则是指定节点的父节点。根节点是唯一没有父节点的节点
同级节点 siblings:如果两个节点拥有相同的父节点,则它们是同级节点
子树和子树根节点 :以节点为根的子树是由以节点为根的节点的后代生成的树
树与节点的基本表示 对于树,最重要的是表现出其节点。通过存储根节点,我们就实际上存储了树
1 2 3 4 5 class TreeNode { Object element ; TreeNode firstChild ; TreeNode nextSibling; }
可以用以下代码来表示树和节点(MIEC版,更详细):
1 2 3 4 5 6 7 8 9 10 11 12 13 14 15 16 17 18 19 20 21 22 public class BinarySearchTree <K extends Comparable <K>, V> { private Node root; private class Node { private K key; private V value; private Node leftChild, rightChild; public Node (K key, V value) { this .key = key; this .value = value; } } public void insert (K key, V value) { ... } public void delete (K key) { ... } public void max ( ) { ... } public void min ( ) { ... } public void successor (K key) { ... } public void predecessor (K key) { ... } public void search (K key) { ... } public void inOrderWalk ( ) { ... } ... }
二叉树 Binary Tree 代码实现部分见“二叉树及相关功能实现”
二叉树是在有限的节点集上定义的结构,这些节点集要么不包含节点,要么由三个不相交的节点集组成:根节点 、称为左子树 的二叉树和称为右子树 的二叉树
Positional Trees and k-ary Tree
k-ary Tree 中的内节点(即不是叶节点的节点)数量
二叉搜索树 Binary Search tree BST 二叉搜索树(BST)是一种二叉树,其中每个节点都有一个可比较的键(和一个关联值),并满足以下限制:任何节点中的键大于该节点左子树中所有节点中的键,小于该节点右子树中所有节点中的键
或者说,对于某个节点对应的值而言,这个值总是大于其左边的值而小于其右边的值
“A binary search tree (BST) is a binary tree where each node has a Comparable key (and an associated value) and satisfies the restriction that the key in any node is larger than the keys in all nodes in that node’s left subtree and smaller than the keys in all nodes in that node’s right subtree.”
树的遍历(基本介绍) 这里基本提一下树的三种遍历方式,详细的代码实现和介绍,于本文《细说树的遍历》一部分
我们前面说过,我们的树自然是使用递归来生成/操作的,自然,想要遍历一棵树,最先想到的也是利用递归 。根据目的和实现,我们最常使用三种遍历:先序遍历、中序遍历、后序遍历
先序遍历:先处理当前节点,再处理两棵子树 中序遍历:依次序列出各项,处理左子树、当前节点、右子树 后序遍历:先处理两棵子树,再处理当前节点
BST:In-Order 按照Left->Root->Right 的顺序输出结果
我们可以利用递归来实现这种搜索,核心的伪代码如下:
1 2 3 4 5 6 7 8 9 10 11 inOrderWalk(currentRoot){ if (currentRoot == NIL) { return ; }else { inOrderWalk(currentRoot.leftChild); print(currentRoot.key) ; inOrderWalk(currentRoot.rightChild); } }
核心部分在于else
部分,我们逐步分解:
1 2 3 4 5 6 7 8 9 10 11 12 13 14 15 16 17 18 19 20 21 22 23 ```print currentRoot.key ;```:在完成一次深度搜索之后,会是前一句```inOrderWalk(currentRoot.leftChild);```的后一句,则可以实现打印,此时打印的结果自然就是刚刚第一个完成搜索得到的点 ```inOrderWalk(currentRoot.rightChild);```:同理。在完成一次左向搜索后,进行右向搜索,然后自然又会进入到一波递归中去 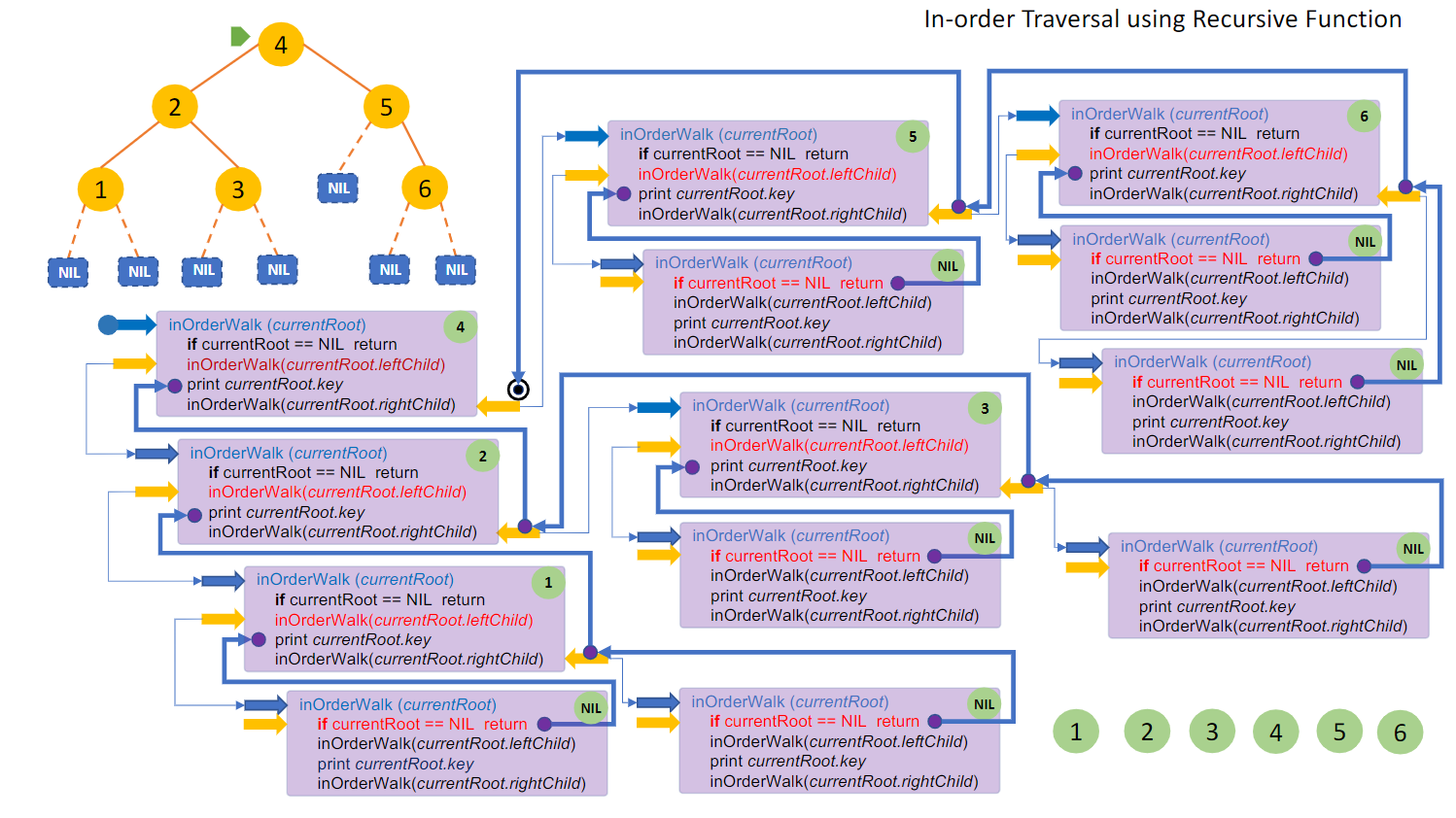 我们同样还可以使用迭代来完成这种搜索,如下: ```Java inOrderWalk(currentRoot){ S = ∅ while S ≠ ∅ or currentRoot ≠ NIL{ if currentRoot ≠ NIL{ //如果还没找到NIL,就是还未触底,就一直向左找并压入栈 PUSH(S, currentRoot) currentRoot = currentRoot.leftChild }else{ //触底后进行一次弹出,并且打印,然后开始向右搜索 currentRoot = POP(S) print currentRoot.key currentRoot = currentRoot.rightChild } } }
可以想象:在首次压栈的过程中,我们不断加入了最左边的一排结点,触底后,我们开始出栈并打印值,此时实际上是从下到上 再次进行了一次搜索,不过这次的目的是打印值与向右搜索。如此反复,则可以得到结果。 核心原理和递归的做法是一样的
BST:Pre-order 同样有两种搜索法,思想和做法同上,只是打印顺序不同:
BST:Post-order 同样有两种搜索法,思想和做法同上,只是打印顺序不同:
小结 对于上述的三种搜索法,我们可以归纳为: 递归:
1 2 3 4 5 6 7 8 9 10 11 12 Methond(当前节点){ 若为空返回 若不为空,则{ A.打印当前 B.左搜索 C.右搜索 上述ABC按要求排序,如对于in-Order,则是BAC(左,当前,右) 对于pre-order则是 ABC(当前,左,右) } }
迭代: 迭代都是利用栈进行,因此和递归法大同小异:
1 2 3 4 5 6 7 8 9 10 11 12 Methon(当前节点){ 如果不为空{ 压入 按需搜索 } 如果为空{ 出栈 打印 按需搜索 } }
在这里和递归相比,各模式的实现的差别较大,建议分别阅读
Level-order Traversal 如果依照层次(即深度)来完成搜索,则要利用到队列
Level-order按水平顺序,从上到下打印
1 2 3 4 5 6 7 8 9 10 11 12 13 14 15 16 17 18 19 levelOrderWalk (currentRoot){ Q = ∅ ENQUEUE(Q, currentRoot) //首先另队列为空,然后让该节点入队 while Q ≠ ∅{ //如果队列不是空的,按下执行操作 currentNode = DEQUEUE(Q) print currentNode.key //首先让当前节点出队,并打印 //然后按照左右节点的情况分别让对应的节点入队 //这里我们按照先左后右的顺序,以此达到了水平顺序遍历的目的 if currentNode.leftChild ≠ NIL ENQUEUE(Q, currentNode.leftChild ) if currentNode.rightChild ≠ NIL ENQUEUE(Q, currentNode.rightChild ) } }
二叉树及相关功能实现(源于书本) 基础与节点定义 同最基本的树的节点,但我们根据二叉树的性质定义了专门的变量
1 2 3 4 5 class BinaryNode { Object element ; BinaryNode left ; BinaryNode right ; }
利用泛型,做出更普遍性的定义:
1 2 3 4 5 6 7 8 9 10 11 12 13 14 15 16 private static class BinaryNode { AnyType element ; BinaryNode<AnyType> left ; BinaryNode<AnyType> right ; BinaryNode(AnyType theElement){ this (theElement,null ,null ); } BinaryNode(AnyType theElement ,BinaryNode<AnyType> lt , BinaryNode<AnyType> rt ){ element = theElement ; left = lt ; right = rt ; } }
后续都会以泛型版本的来写方法,主要使用compareTo
方法,而且开销也会来源于此。这些是后话了
二叉树的基本方法 插入方法insert 1 2 3 4 5 6 7 8 9 10 11 12 13 14 15 16 17 18 19 private BinaryNode<AnyType> insert (AnyType x , BinaryNode<AnyType> t) { if (t == null ) return new BinaryNode <>(x , null , null ); int compareResult = x.compareTo(t.element) if (compareResult < 0 ){ t.left = insert(x,t.left); }else if (compareResult > 0 ){ t.right = insert(x,t.right); }else { } return t ; }
是否有某个值:contains 1 2 3 4 5 6 7 8 9 10 11 12 13 14 15 16 17 18 19 20 21 22 23 24 25 26 private boolean contains (AnyType x , BinaryNode<AnyType> t ) { if (t == null ){ return false ; } int compareResult = x.compareTo(t.element) ; if (compareResult < 0 ){ return contains(x,t.left) ; }else if (compareResult > 0 ){ return contains(x,t.right) ; }else { return true ; } }
最值查找findMin/findMax 其二,我们需要知道如何得到最值,事实上这些内容都大同小异: 包含两种方法递归和非递归实现,以左树为递归例子,右树为非递归例子 在树中的查找,实际上是不比较值的,找小的就一直往左到底部,找大的同理 因为值的比较,实际上是在生成树的时候就进行的了
1 2 3 4 5 6 7 8 9 10 11 12 13 14 15 16 17 18 19 20 21 22 23 24 25 private BinaryNode<AnyType> findMin (BinaryNode<AnyType>) { if (t == null ){ return null ; }else if (t.left == null ){ return t ; } return t.findMin(t.left) ; } private BinaryNode<AnyType> findMax (BinaryNode<AnyType>) { if (t != null ){ while (t.right != null ){ t = t.right ; } return t ; } }
二叉查找树的基础实现
删除方法remove 删除反而是普通二叉树中最麻烦的事情,因此单独拿出来说 一般认为有惰性删除与完全删除 惰性删除:把要去除的元素标记为,但是实质并没有把它从树中移除,这种方法除了偷懒之外还有益处,具体为:
二是完全删除,这个方法就是改变了节点之间的连接方式,彻底将元素移出树 理论操作如下,节选自书: 其代码实现如下:
1 2 3 4 5 6 7 8 9 10 11 12 13 14 15 16 17 18 19 20 21 22 23 24 25 26 27 28 29 30 31 32 33 34 35 private BinaryNodes<AnyType> remove (AnyType x,BinaryNode<AnyType> t) { if (t != null ){ return t ; } int compareResult = x.compareTo(t.element) ; if (compareResult < 0 ){ t.left = remove(x,t.left); }else if (compareResult > 0 ){ t.right = remove(x,t.right); }else if (t.left != null && t.right !=null ){ t.element = findMin(t.right).element ; t.right = remove(t.element,t.right) ; }else { t = (t.left != null ) ? t.left:t.right ; } return t ; }
平均情况的算法分析 这部分暂略,表达式的求解在快速排序部分有提及。这里引用书本内容:
二叉树及相关功能实现(源于MIEC) 源于课件伪代码进行的实现,建议看源于书本的部分,更详细且更易懂
最值 在二叉树中,按照其规则,最小值位于最左侧,而最大值位于最右侧,这使得我们可以之间向左/向右 搜索来得到最值
1 2 3 4 findMin(currentRoot) while currentRoot.left ≠ NIL currentRoot = currentRoot.leftChild return currentRoot
1 2 3 4 findMax(currentRoot) while currentRoot.rightChild ≠ NIL currentRoot = currentRoot.rightChild return currentRoot
插入 1 2 3 4 5 6 7 8 9 10 11 12 13 14 15 16 17 18 19 20 21 22 23 24 25 26 27 28 29 Insert(currentRoot,node){ //接受根以及一个节点作为参数 if currentRoot == NIL { return node ; //如果根节点为空,理所当然,我们返回node //实质上的意思就是把它作为根节点 } //下面是根节点非空的情况,我们按照大小顺序来进行位置的插入 if node.key < currentRoot.key{ currentRoot.leftChild = insert(currentRoot.leftChild, node); //如果node的值比根小,就以根的左值为子树的根,再次向下搜索 //这里实际上是用了递归 else if(node.key < currentRoot.key){ //同理,大于则向右 currentRoot.rightChild = insert(currentRoot.leftChild,node); } else{ currentRoot.value = node.value ; } } return currentRoot ; }
删除 首先我们可以分析三种最基础的情况 分别是对应:本来就是叶节点,没有左/右 子树的情况 对应的解法分别是:直接移除该节点,以及移除该节点后将其右/左子树更新到它之前的位置
而当要删除的节点的子树情况比较复杂的时候,我们就需要分别考虑并且更新子节点状态
那么,怎么挑选继承被删除位置node x的点?则继承的点应该是子树中最小 的点,同时也应该是大于x.key 的点
依上分析,我们的代码如下: 我们利用递归来解决该问题:因为实际上,base case和每一步的操作是比较明了的
1 2 3 4 5 6 7 8 9 10 11 12 13 14 15 16 17 18 19 20 public static void delete (currentRoot,key) { if (currentRoot == NIL){ return currentRoot ; } if (key < currentRoot){ currentRoot.leftChild = delete(currentRoot.leftChild,key) ; }else if (key > currentRoot){ currentRoot.rightChild = delete(currentRoot.rightChild,key) ; }else if (currentRoot.leftChild != NIL && currentRoot.rightChild != NIL){ currentRoot.key = findMin(currentRoot.rightChild).key ; currentRoot.rightChild = delete(currentRoot.rightChild , currentRoot.key) ; }else { current = (currentRoot.leftChild != null ) ? currentRoot.leftChild : currentRoot.rightChild ; } return currentRoot ; }